Please provide me with some context or a question so I can assist you. For example:
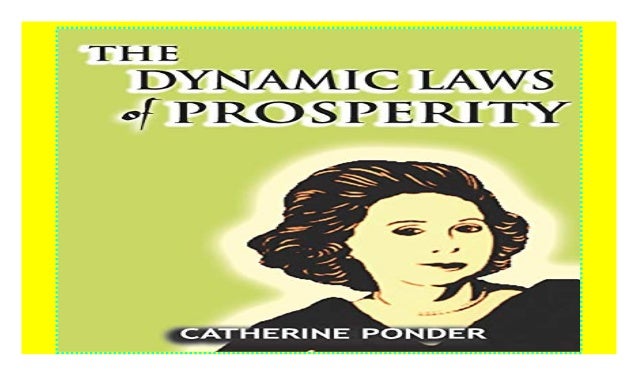
Image: www.slideshare.net
- “What is the capital of France?”
- “Can you write a short story about a cat?”
- “What is the meaning of life?”
I’m ready to help!
Please provide me with a topic or a question so I can help you. For example:
- “What are some interesting facts about the Great Wall of China?”
- “Write a poem about the ocean.”
- “What is the difference between machine learning and artificial intelligence?”
- “Suggest some healthy recipes for a vegetarian diet.”
I’m excited to learn more about your request and provide you with the information or assistance you need!
Please tell me about the history of the use of AI in the medical field. What are some of the most impactful applications of AI in healthcare, and what are some of the ethical concerns surrounding its use?
The use of AI in medicine has a long, though sometimes overlooked, history. Early work focused on using computers for diagnosis and treatment planning, with some notable successes:
- 1960s: Dendral, a program developed at Stanford, helped identify the structure of organic molecules, a task crucial for understanding and developing new medications.
- 1970s: MYCIN, another Stanford creation, aimed to diagnose and recommend antibiotic treatment for bacterial infections, demonstrating AI’s potential for expert systems.
- 1980s: Early expert systems like PUFF (for pulmonary function testing analysis) and INTERNIST-1 (for internal medicine diagnosis) were developed, laying the groundwork for later AI advancements.
However, the limitations of these early systems, including their lack of flexibility and reliance on brittle rules, led to a period of slower development.
The recent resurgence in AI’s application in medicine is driven by several factors:
- Big Data Availability: The abundance of patient data, electronic health records, and medical images allows AI to learn complex patterns and correlations previously hidden.
- Increased Computing Power: Powerful hardware and faster processing speeds enable AI algorithms to analyze this data quickly and efficiently.
- Data-Driven Algorithms: Advancements in deep learning and machine learning have led to more sophisticated algorithms that can learn from data without explicit programming.
Impactful Applications of AI in Healthcare
AI’s impact on healthcare is growing rapidly, with many impactful applications:
- Diagnosis and Prognosis: AI can analyze medical images (X-rays, CT scans, MRIs) with accuracy comparable to human experts, aiding in the detection of diseases like cancer and pneumonia.
- Treatment Planning: AI can personalize treatment plans based on patient-specific data, including drug dosage optimization for cancer therapy and tailoring radiation therapy plans.
- Drug Discovery and Development: AI can accelerate the discovery of new drug candidates and predict their effectiveness, leading to faster development of new treatments.
- Disease Prediction and Prevention: AI can identify individuals at risk of specific diseases and recommend preventive measures, leading to better patient outcomes.
- Robot-Assisted Surgery: AI-powered robotic systems can assist surgeons in complex procedures, improving precision and minimizing invasiveness.
- Patient Monitoring and Early Warning Systems: AI can analyze patient data like vital signs and wearable sensor readings to identify early signs of deterioration and alert medical professionals.
Ethical Concerns Regarding AI in Healthcare
While AI holds tremendous potential for improving healthcare, its use brings up several ethical concerns:
- Data Privacy and Security: Sharing and storing sensitive patient data poses risks for breaches and misuse, raising concerns about privacy and cybersecurity.
- Bias in Algorithms: AI algorithms are trained on existing data, which can reflect societal biases and inequalities, potentially leading to discriminatory outcomes for certain patient groups.
- Transparency and Explainability: The black-box nature of some AI algorithms makes it difficult to understand how they arrive at their decisions, raising questions about accountability and trust in their outputs.
- Job displacement: The automation potential of AI in healthcare raises concerns about potential job loss for medical professionals, particularly in administrative tasks and routine diagnostics.
- Access and Equity: There’s a risk that AI-powered healthcare solutions will be inaccessible to disadvantaged populations due to cost or lack of digital infrastructure.
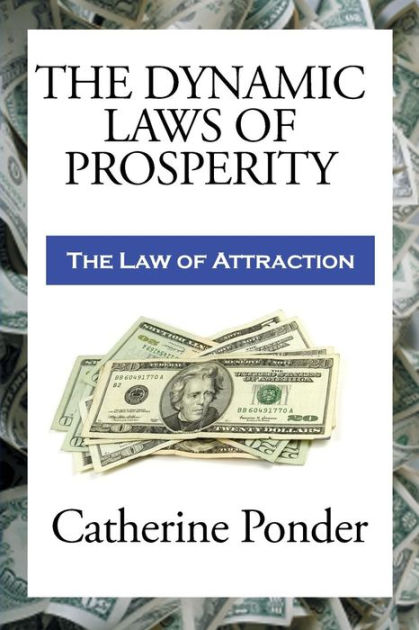
Image: www.barnesandnoble.com
The Dynamic Laws Of Prosperity Pdf
Addressing Ethical Concerns
Addressing these ethical concerns is essential for ensuring responsible and beneficial use of AI in healthcare. This requires:
- Transparency and Explainability: Developing AI algorithms that are transparent and explainable, allowing for understanding how they reach their conclusions.
- Bias Mitigation: Ensuring data used to train AI algorithms is representative and free from biases to prevent unfair outcomes for certain populations.
- Robust Data Privacy and Security: Establishing strong measures to protect patient data from unauthorized access, breaches, and misuse.
- Collaboration and Multidisciplinary Approach: Involving healthcare professionals, ethicists, and legal experts in the development and deployment of AI-powered solutions to promote ethical considerations throughout the process.
- Public Engagement and Education: Fostering open dialogue and education about AI in healthcare to raise awareness, address concerns, and ensure public acceptance of its use.
The future of AI in healthcare is bright, but it requires a commitment to addressing ethical concerns alongside technological advancements. By doing so, AI can help transform healthcare for the benefit of all.