Please provide me with some context or a question so I can assist you.
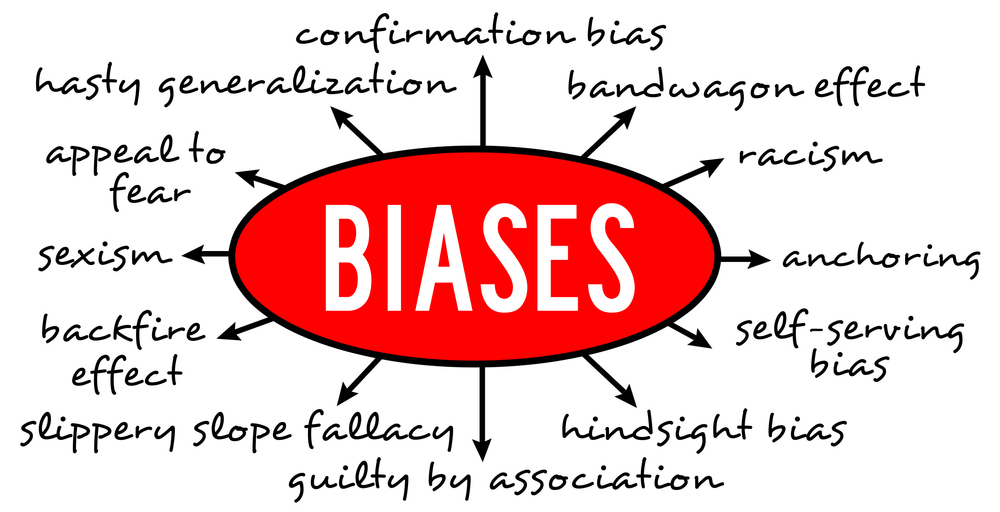
Image: www.thehumancapitalhub.com
For example, you could tell me:
- What you’re working on: “I’m writing a poem about nature.”
- What you need help with: “I need help finding synonyms for ‘beautiful’.”
- A question you have: “What is the capital of France?”
I’m here to help in any way I can!
I’m working on a project where I need to analyze a large dataset of customer reviews about a new product. I’m trying to figure out the most common themes and sentiment expressed in the reviews. Can you help me brainstorm some ways to analyze this data?
Image: hu.linkedin.com
Confronting Bias: Thriving Across Our Differences Free
Here are some approaches for analyzing your customer review dataset:
1. Text Processing & Sentiment Analysis:
- Tokenization and Stop Word Removal: Break down the reviews into individual words (tokens) and remove common words like “the,” “a,” “is,” etc.
- Stemming and Lemmatization: Reduce words to their root form (“running” becomes “run”) for better analysis.
- Part-of-Speech Tagging: Identify the grammatical role of each word (noun, verb, adjective) to gain deeper insights.
- Sentiment Analysis: Use libraries like NLTK or TextBlob to analyze the overall sentiment of each review (positive, negative, neutral).
- Aspect-Based Sentiment Analysis: Identify specific product aspects (e.g., battery life, design) and analyze the sentiment towards each aspect.
2. Theme Extraction & Topic Modeling:
- Keyword Extraction: Identify the most frequent words and phrases in the reviews. Use tools like TF-IDF or RAKE.
- Topic Modeling (LDA, NMF, etc.): Group reviews into clusters based on similar topics. Identify the underlying themes and topics discussed in the reviews.
- Entity Recognition (NER): Identify named entities like brands, products, locations, and dates in the reviews.
- Clustering: Use clustering algorithms (K-Means, DBSCAN) to group reviews based on their similarity. This helps in identifying common patterns and groups of customers.
3. Visualization & Reporting:
- Word Clouds: Visualize the most frequent words in the reviews.
- Sentiment Distribution Graphs: Visualize the overall sentiment distribution of reviews over time.
- Topic Maps: Visualize the relationship between different topics and themes.
- Heatmaps: Show the correlation between specific aspects and customer sentiment.
- Interactive Dashboards: Create a dynamic dashboard to easily visualize key insights and filter data.
4. Additional Considerations:
- Data Cleaning & Preprocessing: Remove duplicates, handle missing data, and address inconsistent formatting.
- Consider External Data Sources: Integrate customer data (e.g., demographic information) with review data to understand customer segments.
- Use Advanced Tools & Libraries: Leverage specialized libraries like SpaCy, Gensim, or Hugging Face for complex tasks like text classification and advanced topic modeling.
- Focus on actionable insights: Translate your analysis into actionable recommendations for product improvement, marketing, or customer service.
Example Scenario:
Imagine you’re analyzing reviews for a new smartwatch. You may find:
- A positive sentiment towards design and battery life.
- Negative sentiment regarding GPS accuracy and app integration.
- A significant number of reviews mentioning difficulties setting up the device.
This analysis can help you understand what’s working well, what needs improvement, and how to target your marketing efforts.
Remember: The best approach depends on your specific goals and the nature of your data. Experiment with different techniques and analyze the results carefully to gain valuable insights.